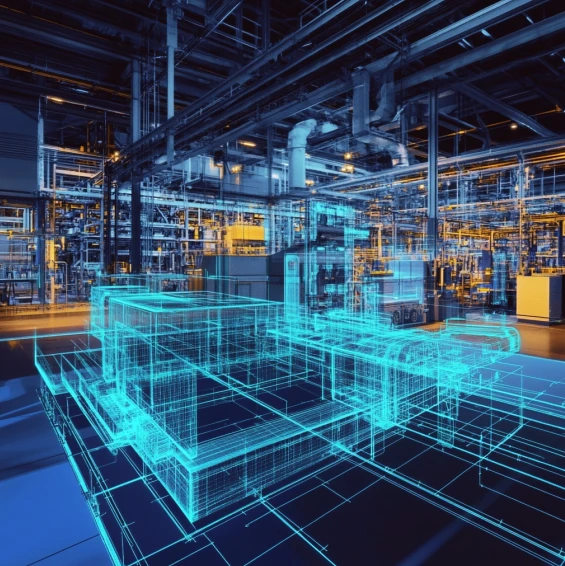
The concept of Digital Twin in the plant simulation environment (Plant Simulation) has become a pillar for process optimization, cost reduction and the promotion of Industry 4.0 in modern manufacturing.
This article will explore in depth what a Digital Twin is in plant simulation, what its essential characteristics are, how it integrates with Industry 4.0, the tangible benefits for companies that implement it, scientific research that supports its effectiveness, case studies, integration challenges, associated costs and the relevance of real-time feedback.
Future projections and the importance of consulting services will also be presented. Download to accelerate the adoption of Digital Twins technologies.
What is Digital Twin in Plant Simulation and why is it relevant?
A Digital Twin in plant simulation (Plant Simulation) is the virtual and accurate representation of a physical manufacturing facility that seeks to reflect the behavior, processes and data of the real environment in real or near real time. The relevance of this virtual model lies in the ability to predict and optimize operations before their physical execution, reducing implementation risks and ensuring efficient use of resources. This technology is supported by the convergence of Industry 4.0, the adoption of IoT sensors and advanced data analytics that provide the opportunity to digitally replicate complete production lines. As an example, an automotive plant that produces 200 vehicles a day can model its assembly stations digitally and simulate different demand scenarios, agilely adjusting its production line and avoiding cost overruns.
The Digital Twin for plant simulation is essential in making informed decisions and optimizing processes, which leads to the following question: What are the main characteristics of a Digital Twin in plant simulation?
What are the main characteristics of a Digital Twin in plant simulation?
For a Digital Twin to be truly effective in plant simulation, it must meet specific criteria that ensure accuracy and usefulness. Below are those essential elements:
- Faithful representation of the virtual model and the real environment: The Digital Twin must include all critical components, from machinery to internal transportation systems, with highly accurate configuration data. The reason for this lies in the need to predict behavior and performance accurately. Example: An electronic components assembly line that includes the speed of each machine and stock turnover in detail.
- Continuous data collection and analysis: A Digital Twin must have a process for receiving information in real time, or at least, with the lowest possible latency. The reason is that synchronization with the physical environment is maintained in this way. Example: IoT sensors that measure temperature and vibration level at each plant station to adjust manufacturing parameters.
- Prediction and optimization capacity: The Digital Twin must allow running “what if” simulations that project production changes or additions of new manufacturing lines. Example: A packaging company that analyzes a new packaging line and simulates increases in demand by 30% with the Digital Twin to anticipate bottlenecks.
- Scalability and modularity: The Digital Twin must be able to grow in complexity or reduce according to the requirements of the process. The reason is adaptability to different plant sizes. Example: Start with a section of the plastic injection plant and then expand to the final assembly section.
- Integration with IT and OT systems: The Digital Twin must connect with ERP, MES and plant control systems to seamlessly exchange data. Example: An MES system that sends production data to the simulation platform, which adjusts planning parameters in real time.
These characteristics provide solid foundations for the relationship with Industry 4.0 in simulation environments.
How is the Digital Twin related to Industry 4.0 in plant simulation environments?
Industry 4.0 integrates key technologies such as cloud computing, IoT, Big Data, Machine Learning and cyber-physical systems to modernize and optimize production. The Digital Twin in plant simulation is an essential component in this strategy by providing a bridge between the physical and the virtual. The reason for this integration is to allow real-time communication and decision making based on reliable data coming from the real plant.
Continuous feedback from IoT devices and big data analysis (Big Data Analytics) enable constant monitoring of the Digital Twin, adjusting the simulation to any fluctuations in the real plant, whether due to raw material delays, machinery failures or changes in market demand. According to a study by the Fraunhofer Institute (2021), companies that integrate Digital Twins in their Industry 4.0 strategy manage to reduce their unplanned downtime by 15%.
Understanding how the Digital Twin supports Industry 4.0 facilitates the discussion about the tangible benefits for companies that implement it.
What benefits do companies obtain by integrating Digital Twin in plant simulation?
The adoption of a Digital Twin in plant simulation entails multiple competitive and economic advantages. The most frequently achieved benefits are listed below:
- Optimization of production processes and cost reduction
- Definition: Minimize rework and downtime through a virtual model that safely tests configurations and adjustments.
- Reason: Anticipate possible problems and iterate before physically carrying them out.
- Example: A metalworking company that, through simulation, reduces energy consumption by 10% by adjusting the oven ignition sequence.
- Risk reduction and quality improvements
- Definition: Identify potential failures in machines and processes before their actual appearance.
- Reason: Promote the implementation of optimal predictive and corrective maintenance.
- Example: A food plant that, with the Digital Twin, detects that the packaging line requires a seal change every 2,500 containers, reducing waste by 20%.
- Flexibility in the face of demand fluctuations
- Definition: Accelerate the ability to respond to sudden increases or decreases in production.
- Reason: Manage installed capacity by virtually seeing the impact of each change on the line.
- Example: A textile factory that simulates a 25% peak demand and adjusts production shifts to meet deadlines without oversizing the workforce.
- Advanced analytics and informed decision making
- Definition: Integrate Big Data tools and Machine Learning algorithms to forecast operating trends.
- Reason: Increase accuracy in planning and forecasting inventory needs.
- Example: Chemical products plant that, through predictive algorithms, reduces shortages by 15% by adjusting raw material orders.
- Improvement in safety and reduction of accidents
- Definition: Simulate dangerous conditions and establish protocols before putting employees at real risk.
- Reason: Have more realistic training scenarios and safety regulations.
- Example: A petrochemical plant that, through simulation, trains personnel in leak emergencies before enabling the new line.
These benefits are supported by evidence from successful case studies in the manufacturing industry.
How is a Digital Twin implemented in plant simulation in a practical way?
Practical implementation requires a systematic approach. Below are the essential steps:
- Define the scope and objectives: Establish what you want to optimize or analyze: inventory, layout or production flows.
- Collect critical plant data: Obtain machine measurements, cycle times, energy consumption and transport routes.
- Select the simulation platform: Choose software compatible with ERP and MES systems, which allows data to be updated in real time.
- Model the virtual plant: Create the structure of stations, machines and flows; calibrate initial parameters.
- Validate and refine the model: Compare simulation results with historical data and adjust until an acceptable correlation is achieved (above 90%).
- Incorporate optimization algorithms: Apply Machine Learning techniques or heuristics to evaluate multiple scenarios.
- Monitor and maintain synchronization: Implement a periodic data update plan and a real-time feedback channel.

In this way, it is ensured that the Digital Twin has relevance and value in daily operations. However, real-time feedback is an essential component that deepens the effectiveness of this technology, so the following question arises: What is the importance of real-time feedback for Digital Twin and plant simulation?
What is the importance of real-time feedback for Digital Twin and plant simulation?
Real-time feedback allows the Digital Twin to continuously receive and process updated data on plant performance. This enables a faster response to deviations and facilitates the generation of early warnings. The reason for its importance is based on the need to synchronize the virtual model with real changes to make timely decisions.
As an example, if a sensor detects overheating in a plastic injection machine, the Digital Twin adjusts the simulation and can recommend lowering production by 5% to avoid major failures. Research from the Polytechnic University of Valencia (2019) shows that real-time feedback, by incorporating predictive algorithms, reduces unexpected periods of downtime by up to 18%.
To further support the relevance of these implementations, it is necessary to review scientific studies that validate the application of the Digital Twin.
What scientific research supports the application of Digital Twins in manufacturing plants?
Numerous research centers and universities have carried out studies demonstrating the effectiveness and potential of Digital Twins. Below is a table that brings together some notable contributions:
Institution / Year | Research Focus | Main Finding |
WITH (2020) | Cybersecurity analysis in Digital Twins for manufacturing | Proposes specific security protocols, reducing incidents by 25% in industrial networks. |
Fraunhofer Institute (2021) | Integration of Digital Twin with collaborative robotics | 15% increase in productivity and 10% reduction in accidents by automating work lines. |
Polytechnic University of Valencia (2019) | Implementation of predictive algorithms in plant Digital Twin | Reduction of unexpected downtime by 18% through monitoring and machine learning. |
University of Cambridge (2018) | Modeling of logistics flows with Digital Twin | Optimization of 12% in delivery times and stock reduction by 7%. |
These scientific works illustrate the benefits of the technology and, in turn, point out difficulties that may arise.
What challenges does the integration of a Digital Twin for plant simulation face?
Adopting a Digital Twin entails technical and organizational barriers. Common challenges include:
- Data availability and quality: If sensor networks or data collection systems are not robust, the virtual model becomes unreliable.
- Resistance to change: Employees and management may be skeptical of new tools. Training and dissemination of positive initial results is crucial.
- Cybersecurity and information protection: Simulation platforms handle sensitive operation data, so there is a risk of attacks or leaks.
- Implementation cost and complexity: Acquiring software, installing sensors and adapting processes involves significant initial investments and deployment times.
- Need for specialized personnel: Interpretation and maintenance of the Digital Twin requires professionals with engineering, analytics and IT skills.
These obstacles require considerations of financial and human resources, so it is appropriate to address the question: What costs and resources are required when implementing Digital Twin in plant simulation?
What costs and resources are required when implementing Digital Twin in plant simulation?
The investments necessary to deploy a Digital Twin in plant simulation vary depending on the scale and complexity of the industry. In general terms, they are contemplated:
- Hardware and network infrastructure
- Definition: Servers, storage, IoT devices and industrial networks.
- Reason: Ensure the capture and processing of large volumes of data.
- Specialized software
- Definition: Simulation and analytics platforms, licenses for support and additional modules.
- Reason: Provide dynamic modeling functionality and connectivity with MES and ERP systems.
- Training and human talent
- Definition: Training of engineers and operators in simulation methodologies, data analysis and maintenance of the Digital Twin.
- Reason: Guarantee the correct operation and continuous improvement of the tool.
- Consulting and external services
- Definition: Technical assistance and support from experts in initial or specific stages.
- Reason: Facilitate integration and solve problems with cutting-edge methodologies.
With these costs in mind, many companies seek the support of specialized consultants to optimize investments and reduce implementation risks.
What future projections exist for Digital Twin and plant simulations in the manufacturing industry?
Digital Twins are expected to become a key part of modern manufacturing, evolving towards even closer integration with Artificial Intelligence, advanced robotics and augmented reality. Many projections suggest that, by 2025, more than 60% of medium and large industrial companies will have implemented at least one Digital Twin in some phase of their value chain.
Another perspective indicates that cloud-based simulation will allow for greater scalability and collaboration among globally distributed teams. In addition, regulatory frameworks for interoperability and security standards in Digital Twins are expected to appear. Finally, the rise of green or sustainable manufacturing will give the Digital Twin a leading role in reducing the carbon footprint.
Some of these specific issues are of particular interest to users, so additional clarifications are provided in the following FAQ section, which provides additional information not addressed in the headings.
Additional FAQ for Digital Twin in Plant Simulation
1. How can you measure the ROI of a Digital Twin in the manufacturing plant?
To measure ROI, the total implementation costs (hardware, software, training and consulting) are compared with the benefits achieved (savings in materials, reduction in downtime, increased productivity). For example, if the total investment is $300,000 and $150,000 is saved in one year, a return on investment is projected to be close to 2 years.
2. Do I need a specialized IT team to maintain the Digital Twin?
Yes, it is advisable to have at least one IT engineer and one industrial engineer who understand network components, sensor connectivity, and data structure. Their role is to ensure that the information flow and simulation remain up to date without interruption.
3. Are there standardized protocols for data communication in a Digital Twin?
Yes, protocols such as OPC UA and MQTT are widely used for communication between IoT devices and simulation platforms. These protocols are considered industry standard for their scalability, security, and compatibility with various systems.
4. Can a Digital Twin help with sustainability on the factory floor?
Yes, by simulating different scenarios, the Digital Twin identifies configurations that reduce energy or waste use. In this way, companies can align sustainability goals with improvements in productive efficiency, optimizing costs and reducing environmental impact.
5. Can the same Digital Twin be reused for another different plant?
Not directly. Although some elements are replicated, it is necessary to adjust the model to the specifications of the new plant, including machinery layout, workflows, production volume and control systems.
6. What level of detail is recommended when creating a Digital Twin in plant simulation?
It depends on the objectives of the project. It is suggested to start with a moderate level of detail that includes critical components and then progressively delve deeper into specific aspects if the analysis requires it. An excessive level of detail without a clear purpose can skyrocket the costs and complexity of the model.
7. How are updates or changes to the physical factory managed to keep the Digital Twin in sync?
A periodic maintenance plan for the model is recommended, including reviews after each significant change to the real plant (for example, the addition of a new workstation). If there is real-time feedback, some updates are generated automatically on the platform, but validation by the responsible team is always necessary.
Contact our specialists:
https://descar.tech/esp/#contacto
Recent Comments